Discover how the Core Alignment Model (CAM) uses a Dynamic Attractor and Semantic Distillation to transform noisy LLM outputs into purpose-driven, ethically sound responses. By filtering through layered adaptive processes, CAM solves key issues like hallucinations, context drift, and ethical misalignment. This innovative AI framework enables high-integrity, contextually aware interactions ideal for real-world applications, ensuring AI aligns with user intent, ethical standards, and dynamic environmental feedback.
CAM as a dynamic attractor operates as a central point of alignment that continuously draws LLM outputs toward a balanced state of purpose, context, and ethical integrity. Unlike static systems, CAM adjusts responsively across its layers—Objective Alignment, Boundary Setting, Pattern Recognition, Real-Time Adjustment, and Ethical Oversight—based on incoming user inputs and environmental changes. This attractor role enables CAM to refine and channel LLM responses iteratively, reducing noise and irrelevant content while progressively improving coherence. In this way, CAM dynamically “pulls” the output toward a stable yet adaptable equilibrium that aligns with user intent and situational context.
By functioning as a dynamic attractor, CAM mitigates common issues like semantic drift and hallucination, providing a consistent framework that adapts in real time. This adaptability makes it uniquely suited for applications where responses need to remain relevant, ethically sound, and aligned with both user and environmental factors, creating a refined end product that balances precision, clarity, and integrity.
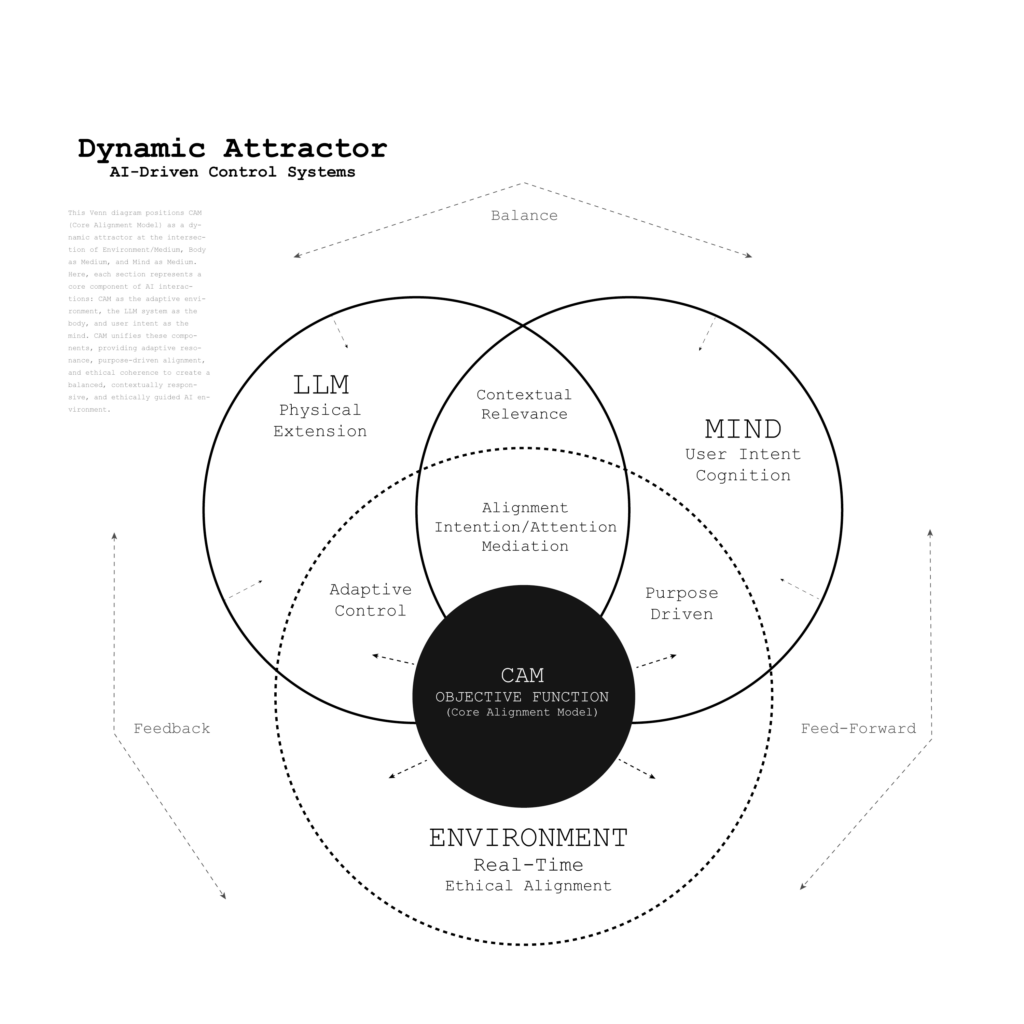
Case:
The research paper focuses on semantics-aware communication systems using mechanisms like Joint Semantics-Noise Coding (JSNC) and Semantic Communication (SSC) to ensure messages retain meaning rather than bit-level accuracy, using distillation and reinforcement learning (RL) for dynamic adaptability. CAM can benefit from this framework by validating its Semantic Distillation process as it also seeks to refine language model outputs through goal-oriented layers. CAM’s ethical and adaptive layers could use RL approaches to better manage noise, semantic drift, and complexity in real-time environments.
Validation and Strengthening CAM’s Case
- Semantic Distillation Parallels: The JSNC’s iterative semantic refinement mirrors CAM’s layered filtration approach, bolstering CAM’s claim as a “semantic distillation filter.” Implementing confidence thresholds for content relevance, as seen in JSNC, could enhance CAM’s adaptive layers for precise contextual alignment.
- Reinforcement Learning Integration: CAM can leverage RL, particularly in its Adaptive Response Mechanism (Tactics) and Values Integration (Ethical Oversight), to adjust in real time while maintaining purpose and ethical coherence. This aligns with SSC’s RL-based, reward-driven communication framework for reducing semantic noise.
- Practical Applications in Real-Time AI: The SSC and JSNC’s focus on practical application in high-noise environments (e.g., dynamic channels) supports CAM’s potential in complex, real-world LLM tasks, where semantic coherence and ethical constraints are critical.
Key Advantages for CAM
This research supports CAM as a highly adaptable framework for generating goal-aligned, ethically coherent AI responses. By integrating these semantic communication principles, CAM can effectively filter LLM outputs, ensuring high relevance, reduced noise, and a balanced trade-off between semantic richness and computational efficiency.
The Dynamic Attractor—represented by the CAM Objective Function—and Semantic Distillation work together to transform noisy, unfiltered LLM outputs into coherent, purpose-aligned responses.
- Dynamic Attractor (CAM Objective Function): Acts as a central guiding force, continuously pulling responses toward alignment with user intent, ethical standards, and contextual clarity. It serves as a goal-oriented anchor, balancing adaptability with purpose-driven outputs.
- Semantic Distillation: Complements the attractor by progressively refining output through layered filtration. Each CAM layer removes irrelevant or misaligned content, enhancing clarity and coherence.
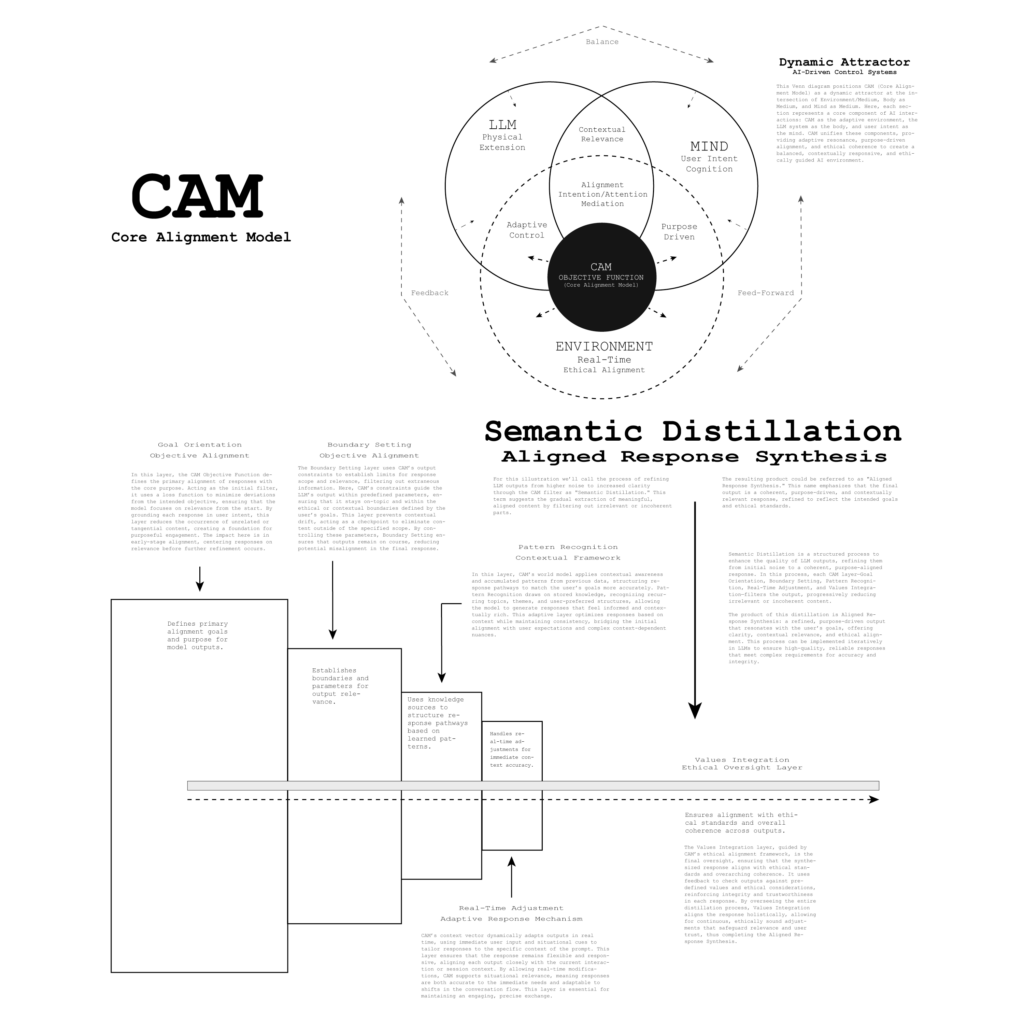
Relationship
As the Dynamic Attractor centers responses around core objectives, Semantic Distillation systematically purifies outputs through Goal Orientation, Boundary Setting, Pattern Recognition, Real-Time Adjustment, and Values Integration. Together, they turn raw, broad LLM responses into precise, ethically sound outputs aligned with real-time user needs.
This dual process enables robust outputs by guiding initial noise through iterative refinement, ensuring each response is dynamically aligned with user intent and adaptive to situational changes.
Together, the Dynamic Attractor and Semantic Distillation form a cohesive system within the CAM framework that allows LLM outputs to move smoothly from broad, raw data states to refined, contextually aligned responses. The Dynamic Attractor (CAM Objective Function) operates continuously, providing a force that keeps the response focused on purpose, context, and ethics, adapting dynamically to changes in user input or situational factors. This adaptability is essential in high-noise environments, where the model’s response may initially contain irrelevant, off-topic, or low-confidence elements.
Semantic Distillation then engages, layering the filtering process in steps that progressively distill information. Each CAM layer (Goal Orientation, Boundary Setting, Pattern Recognition, Real-Time Adjustment, and Values Integration) serves a specific role in refining and filtering the output, ensuring that the response not only aligns with user intent but also remains ethically sound and contextually relevant.
The Dynamic Attractor provides the pull toward alignment, while Semantic Distillation progressively sharpens focus, creating a high-clarity response. As a result, the CAM framework enhances both the quality and integrity of LLM outputs, addressing issues like hallucination, context drift, and ethical misalignment. This dual structure not only improves the reliability of the LLM’s responses but also allows it to adapt to complex real-world applications where situational changes and ethical considerations are critical.
Practical Visualization of the Process
Visualize this system as a concentric layering process:
- The Dynamic Attractor centers the response at the core, setting it on a clear, purpose-driven path.
- Semantic Distillation works outwardly, progressively refining each layer as it moves toward clarity and alignment with the original intent.
This combination ensures that the final response emerges not only as a reliable reflection of user input and purpose but as a flexible, ethically-guided product, capable of adjusting in real-time to shifting conditions in human-machine interactions.
Semantic Distillation in Action
As CAM moves through Semantic Distillation, each layer builds on the refinements of the previous, filtering output toward the aligned response synthesis. This structured process enables precise, ethically sound, and purpose-driven responses, even in complex environments. The process provides several advantages:
- Purposeful Filtering: Each layer acts as a filtration point, ensuring that all content aligns with core goals. This prevents the “drift” often seen in raw LLM responses.
- Contextual Adaptability: With each layer, CAM re-evaluates based on feedback, maintaining focus on the present conversational context. For instance, Real-Time Adjustment dynamically tailors responses in high-stakes environments (e.g., customer service or clinical applications).
- Ethical Integrity and Safety: The Values Integration layer applies ethical coherence across the output, protecting against unwanted or potentially harmful responses. This alignment with ethical guidelines is key for applications in sensitive areas, like healthcare, education, or finance, where trustworthiness and adherence to ethical norms are essential.
Solving Key Challenges in LLM Output
In practical terms, the Dynamic Attractor and Semantic Distillation together solve multiple issues faced by traditional LLMs:
- Hallucinations: By refining outputs through Goal Orientation and Boundary Setting, CAM ensures that the responses generated are both relevant and rooted in real data, significantly reducing hallucinations.
- Context Drift: Semantic Distillation’s adaptive mechanism in Real-Time Adjustment maintains the model’s responsiveness, adjusting outputs to changes in context without losing sight of the primary intent.
- Ethical and Trust Concerns: The conscious layer of Values Integration enables CAM to function with ethical oversight, preserving user trust and aligning responses with defined ethical boundaries.
Practical Implementation and Visualization
To visualize CAM in action, imagine a progressive filtration system where raw responses pass through each CAM layer like a sequence of sieves, each finer and more precise than the last. Initially, the response may contain noise, off-topic ideas, or low-relevance data. The Dynamic Attractor holds the response within a focal pull toward intent alignment, while Semantic Distillation refines and adjusts each layer’s output, discarding unnecessary or irrelevant content and passing only the refined, aligned response onward.
In practical applications, such a structure allows LLMs to work more intuitively with complex, dynamic interactions by bridging human intent with machine processing in real-time. For applications such as virtual assistance, interactive learning platforms, or AI-driven diagnostics, CAM enables outputs that are responsive, relevant, and ethically guided.
Summary: Enabling High-Integrity AI through CAM
The CAM Objective Function, as a Dynamic Attractor combined with Semantic Distillation, offers a solution to the limitations of traditional LLMs, refining outputs to meet high standards of clarity, context-awareness, and ethical coherence. This layered structure allows LLMs to produce consistently reliable, trustworthy, and adaptable responses that better serve human-AI interactions in complex, real-world scenarios.